Mamba: Redefining Sequence Modeling and Outforming Transformers Architecture
Mamba: 시퀀스 모델링 재정의 및 트랜스포머 아키텍처 개선
Published 18 seconds ago on December 18, 2023
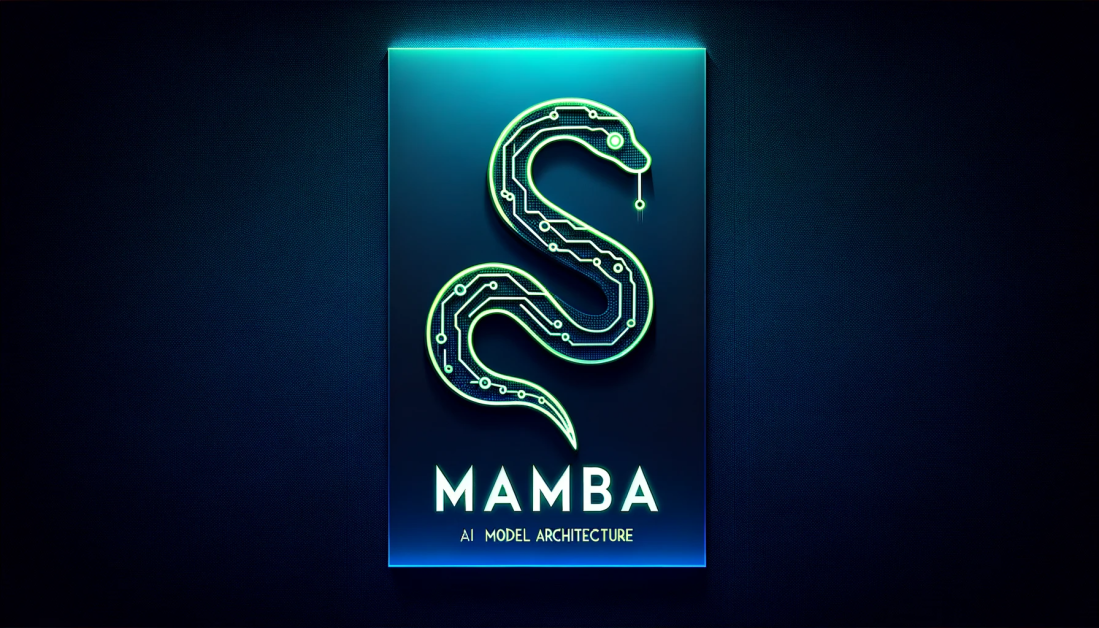
Mamba에 관한 이 글에서는 이 혁신적 상태공간 모델(SSM)이 시퀀스 모델링을 어떻게 혁신하는지 살펴보겠습니다.
Albert Gu와 Tri Dao가 개발한 Mamba는 언어처리, 유전체학, 오디오분석 같은 분야에서 복잡한 시퀀스를 처리하는 효율성이 뛰어납니다.
선택적 상태공간을 사용한 선형시간시퀀스모델링은 이러한 다양한 양식에서 탁월한 성능을 보장합니다.
우리는 특히 긴 시퀀스의 경우 전통적 Transformer가 직면한 계산 문제를 극복하는 Mamba의 능력을 탐구할 것입니다.
상태공간모델의 선택적 접근방식을 통해 추론속도가 빨라지고 시퀀스 길이에 따른 선형확장이 가능해 처리량이 크게 향상됩니다.
Mamba 독창성은 빠른 처리능력, 선택적 SSM레이어, FlashAttention 에서 영감받은 하드웨어 친화적 디자인에 있습니다.
이러한 기능을 통해 Mamba는 변환기 접근방식을 기반으로 한 모델을 포함하여 많은 기존 모델보다 성능이 뛰어나 기계학습 분야에서 주목할 만한 발전을 이루었습니다.
In this article on Mamba, we'll explore how this innovative state-space model (SSM) revolutionizes sequence modeling. Developed by Albert Gu and Tri Dao, Mamba is distinguished for its efficiency in processing complex sequences in fields like language processing, genomics, and audio analysis. Its linear-time sequence modeling with selective state spaces ensures exceptional performance across these diverse modalities.
We'll delve into Mamba's ability to overcome computational challenges faced by traditional Transformers, especially with long sequences. Its selective approach in state space models allows for faster inference and linear scaling with sequence length, significantly improving throughput.
Mamba's uniqueness lies in its rapid processing capability, selective SSM layer, and hardware-friendly design inspired by FlashAttention. These features enable Mamba to outperform many existing models, including those based on the transformer approach, making it a noteworthy advancement in machine learning.
Transformers vs Mamba
GPT-4 같은 Transformer는 자연어처리 분야에서 벤치마크를 설정했습니다.
그러나 시퀀스가 길어지면 효율성이 떨어집니다.
Mamba는 긴 시퀀스를 보다 효율적으로 처리하는 능력과 전체프로세스를 단순화하는 고유한 아키텍처를 통해 앞서 나가고 있습니다.
언어모델의 텍스트와 같은 데이터 시퀀스를 처리하는 데 능숙한 변환기입니다.
데이터를 순차적으로 처리했던 이전 모델과 달리 Transformers는 전체 시퀀스를 동시에 처리하므로 데이터 내의 복잡한 관계를 포착할 수 있습니다.
그들은 예측을 할 때 모델이 시퀀스의 다른 부분에 집중할 수 있도록 하는 주의 메커니즘을 사용합니다.
이 관심은 입력 데이터에서 파생된 쿼리, 키, 값의 3가지 가중치 세트를 사용하여 계산됩니다.
시퀀스의 각 요소는 다른 모든 요소와 비교되어 시퀀스의 다음 요소를 예측할 때 각 요소가 받아야 하는 중요성 또는 '주의'를 나타내는 가중치를 제공합니다.
Transformers, like GPT-4, have set benchmarks in natural language processing. However, their efficiency dips with longer sequences. Here's where Mamba leaps ahead, with its ability to process long sequences more efficiently and its unique architecture that simplifies the entire process.
Transformers adept at handling sequences of data, such as text for language models. Unlike previous models that processed data sequentially, Transformers process entire sequences simultaneously, enabling them to capture complex relationships within the data.
They use attention mechanism, which allows the model to focus on different parts of the sequence when making predictions.
This attention is computed using three sets of weights: queries, keys, and values, derived from the input data. Each element in a sequence is compared to every other element, providing a weight that signifies the importance, or ‘attention', that each element should receive when predicting the next element in the sequence.
Transformer는 입력 데이터를 처리하는 인코더와 출력을 생성하는 디코더라는 2가지 주요 블록을 유지합니다.
인코더는 여러 레이어로 구성되며, 각 레이어에는 다중 헤드 자체 주의 메커니즘과 단순하고 위치별로 완전히 연결된 피드포워드 네트워크라는 2개의 하위레이어가 포함되어 있습니다.
정규화 및 잔차 연결은 각 하위계층에서 사용되어 심층 네트워크 훈련에 도움이 됩니다.
Transformers maintain two main blocks: the encoder, which processes the input data, and the decoder, which generates the output. The encoder consists of multiple layers, each containing two sub-layers: a multi-head self-attention mechanism and a simple, position-wise fully connected feed-forward network. Normalization and residual connections are used at each sub-layer to help in training deep networks.
디코더에는 인코더와 유사한 2개의 하위계층이 있는 레이어도 있지만 인코더의 출력에 대해 다중 헤드 주의를 수행하는 세 번째 하위계층을 추가합니다.
디코더의 순차적 특성으로 인해 위치에 대한 예측은 자동 회귀 속성을 유지하면서 이전 위치만 고려할 수 있습니다.
The decoder also has layers with two sub-layers similar to the encoder but adds a third sub-layer that performs multi-head attention over the encoder's output. The sequential nature of the decoder ensures that predictions for a position can only consider earlier positions, preserving the autoregressive property.
Transformers와 달리 Mamba 모델은 다른 접근 방식을 취합니다.
Transformers는 더 복잡한 Attention 메커니즘을 사용하여 긴 시퀀스 문제를 처리하는 반면 Mamba는 선택적 상태 공간을 사용하여 더 많은 컴퓨팅을 제공합니다.
In contrast to Transformers, the Mamba model takes a different approach. While Transformers deal with the issue of long sequences by using more complex attention mechanisms, Mamba uses selective state spaces, providing a more comput
다음은 변환기의 작동방식에 대한 높은 수준의 개요:
Here's a high-level overview of how a transformer functions:
Input Processing 입력 처리: 변환기는 먼저 입력 데이터를 모델이 이해할 수 있는 형식으로 인코딩하며, 종종 시퀀스의 각 요소 위치도 통합하는 임베딩을 사용 Transformers first encode input data into a format that the model can understand, often using embeddings that also incorporate the position of each element in the sequence.
Attention Mechanism 주의 메커니즘: 기본적으로 주의 메커니즘은 현재 요소를 이해할 때 입력 시퀀스의 다른 부분에 얼마나 집중해야 하는지를 나타내는 점수를 계산. At its core, the attention mechanism computes a score that represents how much focus to put on other parts of the input sequence when understanding a current element.
Encoder-Decoder Architecture: 변환기모델은 입력을 처리하는 인코더와 출력을 생성하는 디코더로 구성. 각각은 입력에 대한 모델의 이해를 구체화하는 여러 레이어로 구성. The transformer model is composed of an encoder to process the input and a decoder to generate the output. Each consists of multiple layers that refine the model's understanding of the input.
Multi-Head Attention: 인코더와 디코더 내에서 멀티 헤드 어텐션을 통해 모델은 다양한 표현 공간에서 시퀀스의 다양한 부분에 동시에 주의를 기울일 수 있어 다양한 컨텍스트에서 학습하는 능력이 향상됩니다. Within both the encoder and decoder, multi-head attention allows the model to simultaneously attend to different parts of the sequence from different representational spaces, improving its ability to learn from diverse contexts.
Position-wise Feed-Forward Networks: 위치별 피드포워드 네트워크: 주의를 기울인 후 간단한 신경망은 각 위치의 출력을 개별적으로 동일하게 처리합니다. 이는 잔여 연결을 통해 입력과 결합된 후 레이어 정규화가 수행됩니다. After attention, a simple neural network processes the output of each position separately and identically. This is combined with the input through a residual connection and followed by layer normalization.
Output Generation 출력 생성: 그런 다음 디코더는 인코더의 컨텍스트와 지금까지 생성된 내용의 영향을 받아 출력 시퀀스를 예측합니다. The decoder then predicts an output sequence, influenced by the encoder's context and what it has generated so far.
시퀀스를 병렬로 처리하는 변환기의 능력과 강력한 주의 메커니즘은 번역 및 텍스트생성 같은 작업에 강력합니다.
대조적으로, Mamba 모델은 선택적 상태공간을 사용하여 시퀀스를 처리함으로써 다르게 작동합니다.
이 접근방식은 긴 시퀀스를 처리할 때 Transformer의 계산 비효율성을 해결합니다.
The transformer’s ability to handle sequences in parallel and its robust attention mechanism make it powerful for tasks like translation and text generation.
In contrast, the Mamba model operates differently by using selective state spaces to process sequences. This approach addresses the computational inefficiency in Transformers when dealing with lengthy sequences.
Mamba의 디자인은 더 빠른 추론을 가능케 하고 시퀀스 길이에 따라 선형적으로 확장하여 특히 시퀀스가 점점 길어짐에 따라 더 효율적일 수 있는 시퀀스모델링을 위한 새로운 패러다임을 설정합니다.
Mamba's design enables faster inference and scales linearly with sequence length, setting a new paradigm for sequence modeling that could be more efficient, especially as sequences become increasingly lengthy.
https://smartstore.naver.com/dopza/products/4569179898
Mamba
Mamba를 정말 독특하게 만드는 것은 전통적인 주의 및 MLP 블록에서 벗어났다는 것입니다.
이러한 단순화로 인해 시퀀스 길이에 따라 선형적으로 확장되는 더 가볍고 빠른 모델이 탄생했습니다.
이는 이전 모델과 비교할 수 없는 성과입니다.
What makes Mamba truly unique is its departure from traditional attention and MLP blocks. This simplification leads to a lighter, faster model that scales linearly with the sequence length – a feat unmatched by its predecessors.
Key features of Mamba include:
Mamba의 주요 기능:
선택적 SSM: 이를 통해 Mamba는 관련 없는 정보를 필터링하고 관련 데이터에 집중하여 시퀀스 처리를 향상할 수 있습니다. 이러한 선택성은 효율적인 내용 기반 추론에 매우 중요합니다. Selective SSMs: These allow Mamba to filter irrelevant information and focus on relevant data, enhancing its handling of sequences. This selectivity is crucial for efficient content-based reasoning.
하드웨어 인식 알고리즘: Mamba는 최신 하드웨어, 특히 GPU에 최적화된 병렬 알고리즘을 사용합니다. 이 설계를 통해 기존 모델에 비해 더 빠른 계산이 가능하고 메모리 요구 사항이 줄어듭니다. Hardware-aware Algorithm: Mamba uses a parallel algorithm that's optimized for modern hardware, especially GPUs. This design enables faster computation and reduces the memory requirements compared to traditional models.
단순화된 아키텍처: Mamba는 선택적 SSM을 통합하고 주의 및 MLP 블록을 제거함으로써 더 간단하고 동질적인 구조를 제공합니다. 이로 인해 확장성과 성능이 향상됩니다. Simplified Architecture: By integrating selective SSMs and eliminating attention and MLP blocks, Mamba offers a simpler, more homogeneous structure. This leads to better scalability and performance.
Mamba는 언어, 오디오, 유전체학 등 다양한 영역에서 탁월한 성능을 입증했으며 사전훈련과 영역별 작업 모두에서 탁월합니다.
예를 들어, 언어 모델링에서 Mamba는 더 큰 Transformer 모델의 성능과 일치하거나 그 이상입니다.
Mamba의 코드와 사전훈련된 모델은 GitHub에서 커뮤니티에서 공개적으로 사용할 수 있습니다.
Mamba has demonstrated superior performance in various domains, including language, audio, and genomics, excelling in both pretraining and domain-specific tasks. For instance, in language modeling, Mamba matches or exceeds the performance of larger Transformer models.
Mamba's code and pre-trained models are openly available for community use at GitHub.
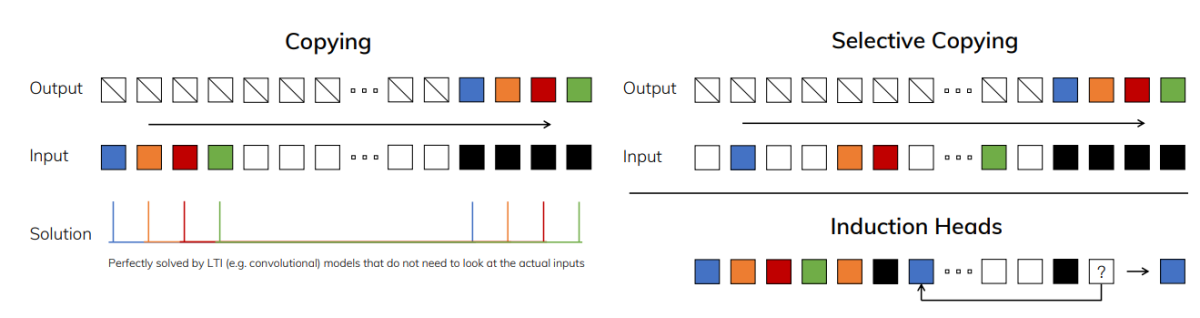
선형 모델의 경우 표준 복사 작업이 간단합니다. 선택적 복사 및 인덕션 헤드에는 LLM을 위한 동적 콘텐츠 인식 메모리가 필요합니다.
Standard Copying tasks are simple for linear models. Selective Copying and Induction Heads require dynamic, content-aware memory for LLMs.
구조화된 상태공간(S4) 모델은 최근 RNN, CNN 및 기존 상태공간모델 특성을 포함하는 유망한 시퀀스모델 클래스로 등장했습니다.
S4 모델은 연속 시스템, 특히 암시적 잠재 상태를 통해 1차원 기능 또는 시퀀스를 매핑하는 시스템 유형에서 영감을 얻습니다.
딥러닝 맥락에서 이는 효율적이고 적응성이 뛰어난 시퀀스모델을 설계하기 위한 새로운 방법론을 제공하는 중요한 혁신을 나타냅니다.
Structured State Space (S4) models have recently emerged as a promising class of sequence models, encompassing traits from RNNs, CNNs, and classical state space models. S4 models derive inspiration from continuous systems, specifically a type of system that maps one-dimensional functions or sequences through an implicit latent state. In the context of deep learning, they represent a significant innovation, providing a new methodology for designing sequence models that are efficient and highly adaptable.
The Dynamics of S4 Models
S4 모델의 역동성
SSM (S4) 기본 구조화된 상태 공간 모델입니다.
시퀀스 x를 취하고 학습된 매개변수 A, B, C와 지연 매개변수 Δ를 사용하여 출력 y를 생성합니다.
변환에는 매개변수를 이산화하고(연속함수를 이산함수로 전환) 시간 불변인 SSM 연산을 적용하는 작업이 포함됩니다 - 즉, 다른 시간 단계에 따라 변경되지 않습니다.
SSM (S4) This is the basic structured state space model. It takes a sequence x and produces an output y using learned parameters A, B, C, and a delay parameter Δ. The transformation involves discretizing the parameters (turning continuous functions into discrete ones) and applying the SSM operation, which is time-invariant—meaning it doesn't change over different time steps.
The Significance of Discretization
이산화의 중요성
이산화는 고정된 공식을 통해 연속 매개변수를 이산 매개변수로 변환하여 S4 모델이 연속시간 시스템과의 연결을 유지할 수 있도록 하는 핵심 프로세스입니다.
이는 모델에 해상도 불변성과 같은 추가 속성을 부여하고 적절한 정규화를 보장하여 모델 안정성과 성능을 향상시킵니다.
또한 이산화는 네트워크를 통한 정보흐름을 관리하는 데 중요한 RNN의 게이팅 메커니즘과 유사합니다.
Discretization is a key process that transforms the continuous parameters into discrete ones through fixed formulas, enabling the S4 models to maintain a connection with continuous-time systems. This endows the models with additional properties, such as resolution invariance, and ensures proper normalization, enhancing model stability and performance. Discretization also draws parallels to the gating mechanisms found in RNNs, which are critical for managing the flow of information through the network.
Linear Time Invariance (LTI)
선형 시불변(LTI)
S4 모델의 핵심 기능은 선형 시불변입니다.
이 속성은 모델의 역학이 모든 시간 단계에 대해 고정된 매개변수를 사용하여 시간이 지나도 일관되게 유지된다는 것을 의미합니다.
LTI는 반복 및 컨볼루션의 초석으로, 시퀀스 모델 구축을 위한 간단하면서도 강력한 프레임워크를 제공합니다.
A core feature of the S4 models is their linear time invariance. This property implies that the model’s dynamics remain consistent over time, with the parameters fixed for all timesteps. LTI is a cornerstone of recurrence and convolutions, offering a simplified yet powerful framework for building sequence models.
Overcoming Fundamental Limitations
근본적 한계를 극복하다
S4 프레임워크는 전통적으로 LTI 특성으로 인해 제한되어 왔으며 이는 적응형 역학이 필요한 데이터 모델링에 어려움을 안겨주었습니다.
최근 연구 논문에서는 시간에 따라 변하는 매개변수를 도입하여 LTI의 제약을 제거함으로써 이러한 한계를 극복하는 접근방식을 제시합니다.
이를 통해 S4 모델은 더욱 다양한 시퀀스와 작업세트를 처리하여 적용 가능성을 크게 확장할 수 있습니다.
'상태공간모델'이라는 용어는 잠재 상태와 관련된 모든 반복 프로세스를 광범위하게 다루며 여러 분야에 걸쳐 다양한 개념을 설명하는 데 사용되었습니다.
딥 러닝의 맥락에서 S4 모델 또는 구조화된 SSM은 복잡한 시퀀스를 모델링하는 기능을 유지하면서 효율적인 계산을 위해 최적화된 특정 클래스의 모델을 나타냅니다.
S4 모델은 엔드투엔드 신경망 아키텍처에 통합되어 독립형 시퀀스 변환 기능을 수행할 수 있습니다.
이는 다양한 신경망 아키텍처에서 시퀀스 모델링을 위한 백본을 제공하는 CNN의 컨볼루션 레이어와 유사하다고 볼 수 있습니다.
The S4 framework has been traditionally limited by its LTI nature, which poses challenges in modeling data that require adaptive dynamics. The recent research paper presents a approach that overcomes these limitations by introducing time-varying parameters, thus removing the constraint of LTI. This allows the S4 models to handle a more diverse set of sequences and tasks, significantly expanding their applicability.
The term ‘state space model' broadly covers any recurrent process involving a latent state and has been used to describe various concepts across multiple disciplines. In the context of deep learning, S4 models, or structured SSMs, refer to a specific class of models that have been optimized for efficient computation while retaining the ability to model complex sequences.
S4 models can be integrated into end-to-end neural network architectures, functioning as standalone sequence transformations. They can be viewed as analogous to convolution layers in CNNs, providing the backbone for sequence modeling in a variety of neural network architectures.
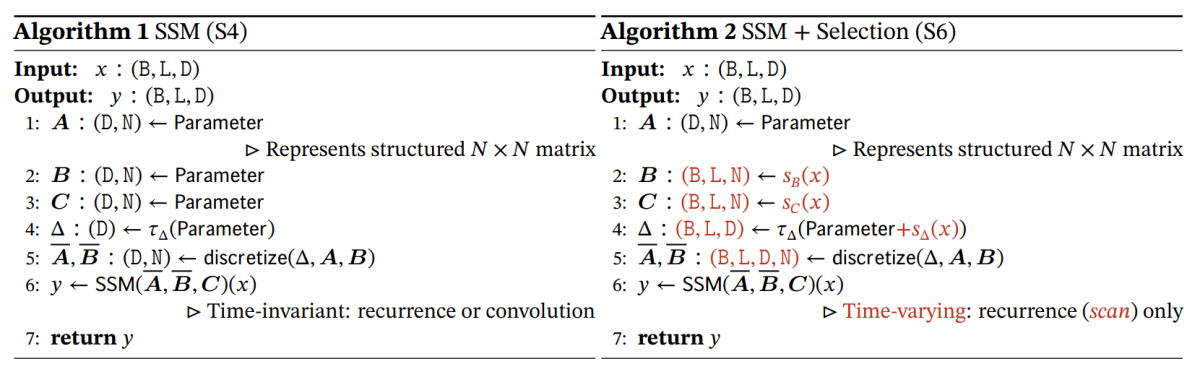
SSM vs SSM + Selection
Motivation for Selectivity in Sequence Modeling
시퀀스 모델링의 선택성에 대한 동기
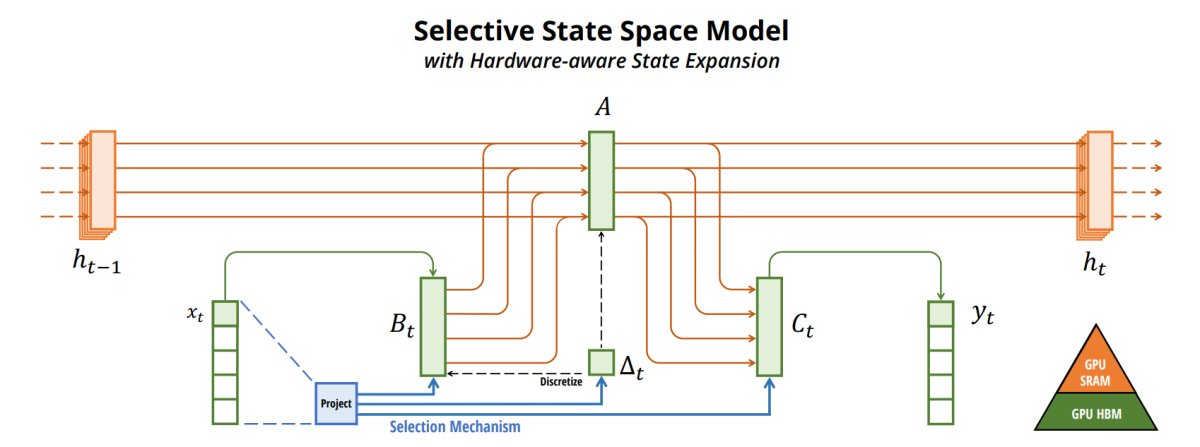
구조화된 SSM
Structured SSMs
이 논문에서는 시퀀스 모델링의 기본 측면이 컨텍스트를 관리 가능한 상태로 압축하는 것이라고 주장합니다.
입력에 선택적으로 집중하거나 필터링할 수 있는 모델은 이러한 압축 상태를 유지하는 보다 효과적 수단을 제공하여 보다 효율적이고 강력한 시퀀스 모델로 이어집니다.
The paper argues that a fundamental aspect of sequence modeling is the compression of context into a manageable state. Models that can selectively focus on or filter inputs provide a more effective means of maintaining this compressed state, leading to more efficient and powerful sequence models.
이러한 선택성은 모델이 시퀀스 차원을 따라 정보가 흐르는 방식을 적응적으로 제어하는 데 필수적이며, 이는 언어모델링 및 그 이상에서 복잡한 작업을 처리하는 데 필수적인 기능입니다.
선택적 SSM은 매개변수가 입력에 종속되도록 함으로써 기존 SSM을 향상시키며, 이는 이전에는 시불변 모델로는 달성할 수 없었던 적응성을 제공합니다.
이로 인해 효율적인 계산을 위해 더 이상 컨볼루션을 사용할 수 없고 대신 선형 반복 메커니즘에 의존하는 시변 SSM이 생성됩니다. 이는 기존 모델과 상당한 차이가 있습니다.
This selectivity is vital for models to adaptively control how information flows along the sequence dimension, an essential capability for handling complex tasks in language modeling and beyond.
Selective SSMs enhance conventional SSMs by allowing their parameters to be input-dependent, which introduces a degree of adaptiveness previously unattainable with time-invariant models. This results in time-varying SSMs that can no longer use convolutions for efficient computation but instead rely on a linear recurrence mechanism, a significant deviation from traditional models.
SSM + 선택(S6) 이 변형에는 선택 메커니즘이 포함되어 있으며 매개변수 B 및 C에 입력 종속성과 지연 매개변수 Δ를 추가합니다.
이를 통해 모델은 입력 시퀀스 x의 특정 부분에 선택적으로 집중할 수 있습니다.
매개변수는 선택사항을 고려하여 이산화되고 SSM 작업은 요소를 순차적으로 처리하여 시간에 따라 동적으로 초점을 조정하는 스캔 작업을 사용하여 시간에 따라 변하는 방식으로 적용됩니다.
SSM + Selection (S6) This variant includes a selection mechanism, adding input-dependence to the parameters B and C, and a delay parameter Δ. This allows the model to selectively focus on certain parts of the input sequence x. The parameters are discretized taking into account the selection, and the SSM operation is applied in a time-varying manner using a scan operation, which processes elements sequentially, adjusting the focus dynamically over time.
Performance Highlights of Mamba
Mamba의 성능 하이라이트
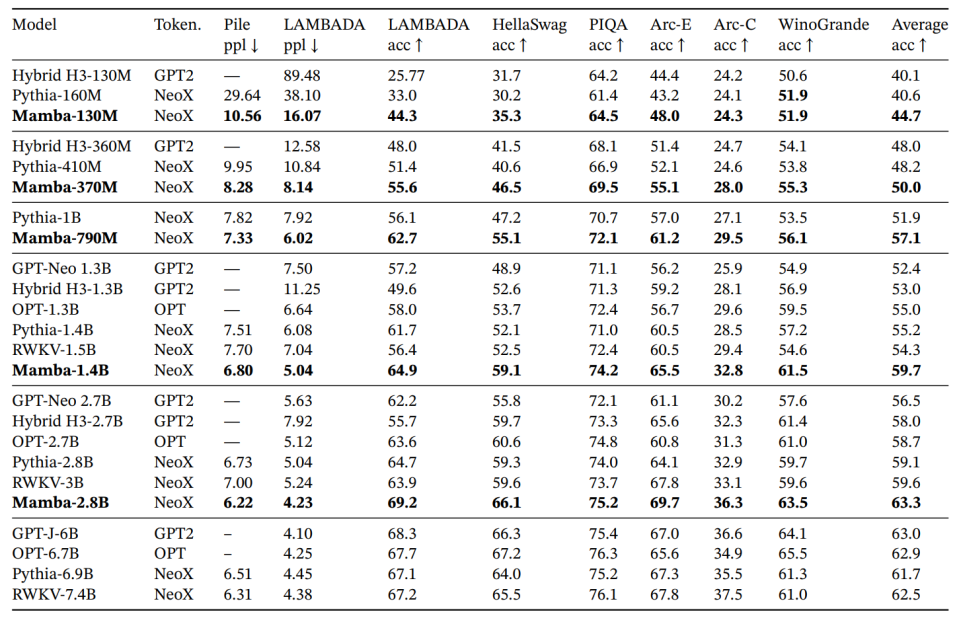
Mamba is best-in-class on every single evaluation result
Mamba는 모든 평가 결과에서 동급 최고입니다.
성능측면에서 Mamba는 추론속도와 정확성 모두에서 뛰어납니다.
이 디자인은 DNA와 오디오 모델링 모두에서 입증된 더 긴 컨텍스트를 더 잘 활용할 수 있도록 하며, 장거리 종속성을 요구하는 복잡한 작업에서 이전 모델보다 성능이 뛰어납니다.
다양한 작업에 대한 제로샷 평가에서도 그 다양성이 강조되어 효율성과 확장성측면에서 이들 모델에 대한 새로운 표준을 설정합니다.
In terms of performance, Mamba excels in both inference speed and accuracy. It's design enables better utilization of longer contexts, which is demonstrated in both DNA and audio modeling, outperforming prior models on complex tasks requiring long-range dependencies. Its versatility is also highlighted in zero-shot evaluations across multiple tasks, setting a new standard for such models in terms of efficiency and scalability.
Getting Started with Mamba
맘바 시작하기
Mamba 활용에 관심이 있는 경우 기술요구사항에는 Linux OS, NVIDIA GPU, PyTorch 1.12+, CUDA 11.6+가 포함됩니다.
설치에는 Mamba 저장소에서 필요한 패키지를 설치하는 간단한 pip 명령이 포함됩니다.
PyTorch 버전에서 호환성 문제가 발생하는 경우 pip와 함께 –no-build-isolation 플래그를 사용하면 도움이 될 수 있습니다.
Pile 및 SlimPajama 데이터세트 같은 광범위한 데이터세트로 훈련된 이러한 모델은 다양한 계산 요구사항과 성능 벤치마크를 충족하도록 설계되었습니다.
Mamba는 선택적 SSM계층부터 Mamba 블록 및 전체 언어모델구조 까지 다양한 수준의 인터페이스를 제공합니다.
아키텍처의 주요 모듈인 Mamba 블록은 인과 Conv1d 레이어를 활용하며 신경망 설계에 쉽게 통합될 수 있습니다.
제공된 Python 사용 예제는 Mamba 모델을 인스턴스화하고 이를 통해 데이터를 처리하는 방법을 보여 주며 시스템의 단순성과 유연성을 강조합니다.
사전훈련된 Mamba 모델은 Hugging Face에서 사용할 수 있으며, 크기는 130M에서 2.8B에 이르는 매개변수이며, 광범위한 Pile 데이터세트와 SlimPajama 데이터세트에서 훈련되었습니다.
이러한 모델은 GPT-3의 차원 표준을 준수하면서 다양한 계산 및 성능 요구사항을 충족하도록 설계되었습니다.
사용자는 이러한 모델에서 높은 처리량과 정확성을 기대할 수 있으므로 Mamba는 언어모델링을 포함하되 이에 국한되지 않는 다양한 애플리케이션에서 경쟁력 있는 선택이 됩니다.
For those interested in leveraging Mamba, the technical requirements include a Linux OS, an NVIDIA GPU, PyTorch 1.12+, and CUDA 11.6+. Installation involves simple pip commands to install the necessary packages from the Mamba repository. If compatibility issues arise with PyTorch versions, using the –no-build-isolation flag with pip can help. These models, trained on extensive datasets like the Pile and the SlimPajama dataset, are designed to meet various computational needs and performance benchmarks.
Mamba offers different levels of interfaces, from the selective SSM layer to the Mamba block and complete language model structures. The Mamba block, which is the architecture's main module, utilizes a causal Conv1d layer and can be easily integrated into neural network designs. The provided usage example in Python demonstrates instantiating a Mamba model and processing data through it, highlighting the simplicity and flexibility of the system.
Pretrained Mamba models are available on Hugging Face, with sizes ranging from 130M to 2.8B parameters, trained on the extensive Pile dataset and the SlimPajama dataset. These models are designed to meet diverse computational and performance requirements, adhering to the dimensional standards of GPT-3. Users can expect high throughput and accuracy from these models, making Mamba a competitive choice for various applications, including but not limited to language modeling.
Mamba's Impact 영향
Mamba는 정보밀도가 높은 데이터를 처리하기 위한 Transformer아키텍처에 대한 강력한 대안을 제공하여 시퀀스 모델링의 도약을 나타냅니다.
그 디자인은 최신 하드웨어의 요구사항에 맞춰 메모리사용과 병렬처리기능을 모두 최적화합니다.
Mamba의 코드베이스와 사전훈련된 모델의 오픈소스 가용성을 통해 Mamba는 AI 및 딥러닝 분야의 연구원과 개발자가 접근할 수 있고 강력한 도구가 됩니다.
Mamba represents a leap forward in sequence modeling, offering a powerful alternative to Transformer architectures for processing information-dense data. Its design aligns with the demands of modern hardware, optimizing both memory usage and parallel processing capabilities. The open-source availability of Mamba's codebase and its pretrained models makes it an accessible and robust tool for researchers and developers in the field of AI and deep learning.
https://amzn.to/3Rvsstd
www.dopza.com
돕자몰 - 강아지 고양이 발작 경련 CBD 오일 전문
반려동물 개 고양이 발작 경련 통증 CBD 오일 전문
www.dopza.com
댓글